No.69
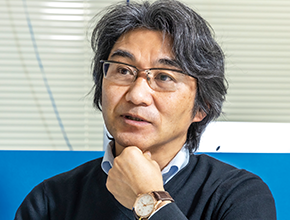
ネットワーク工学の研究
Research on Network Engineering
小さな一つひとつが、大きな全体として知的に振る舞う
スマホやロボットを使ってネットワークの力を応用
Each small thing acts intelligently as part of a greater whole
Applying the power of networks using smartphones and robots
環境都市工学部
滝沢 泰久 教授
Faculty of Environmental and Urban Engineering
Professor Yasuhisa Takizawa
イワシの群れの動きに目を奪われたことはないだろうか。一匹一匹は小さな魚なのに、集団になると一つの生き物のように統制のとれた振る舞いをする。脳も同様に、無数の神経細胞がネットワークを構築して知性を生み出している。このネットワークの力を工学応用しようと考えるのが、環境都市工学部の滝沢泰久教授だ。
Have you ever been amazed by a school of sardines? An individual sardine is small, but upon forming a group, they act together like a single creature. Likewise, in the brain, an infinite number of neurons build a network in a similar way, which creates intelligence. One person who is attempting to apply the power of such a network to engineering is Prof. Yasuhisa Takizawa of the Faculty of Environmental and Urban Engineering.
ネットワークを知能としてとらえる
専門分野について教えてください。
ネットワーク工学です。近年急速に進化している「IoT(モノのインターネット)」「ビッグデータ」「AI」という情報処理の3分野のうちのIoTに関連します。自動車のエンジンや橋脚などさまざまなモノにセンサーと無線機を付け、その情報を無線ネットワークを通してインターネット上に集めるという技術です。
ネットワークには、データを運ぶ伝送路やデータベースといった側面があります。私たちはその先の「ネットワークを知能としてとらえたら、いろいろなことができそうだ」という視点で研究しています。脳は、神経系がネットワークを作ることによって、日々の体験を記憶として蓄えています。魚や鳥の群れも、全体として非常に知的な振る舞いをするネットワークです。
イワシの群れは、捕食者が来たら柔軟に形を変えますね。一つの生き物のようです。
一匹一匹は狭い範囲しか見えていませんが、集まるとあのような構造になります。一つひとつは非常に単純で局所の情報しか得られないのに、それぞれが相互作用して全体で知的な構造を示す。それがネットワークの力です。
Considering a network as intelligence
Tell us about your specialist area of research.
I specialize in Network Engineering. Of the three information processing fields—“Internet of Things (IoT),” “Big Data,” and “AI” —which have been progressing rapidly in recent years, network engineering is related to the IoT. It is a technology that collects information on the Internet through wireless networks by attaching sensors and wireless devices to various things such as automobile engines and bridge piers.
A network has some characteristics such as being a transmission channel for data and a database. Taking a step further, we are conducting research from the viewpoint that "if it were possible to consider a network as intelligence, it would be possible to do a variety of things.” The brain stores daily experiences as memories via networks created by the neural system. A school of fish or a flock of birds is also a network that acts as a whole very intelligently.
A school of sardines changes its shape flexibly when a predator approaches, doesn’t it? It looks like a single creature.
An individual sardine can see only a small area. However, when sardines school up, they form a structure. A sardine is very simple and can get only a limited range of information, but they interact with one another as a whole and display an intellectual structure. That is the power of a network.
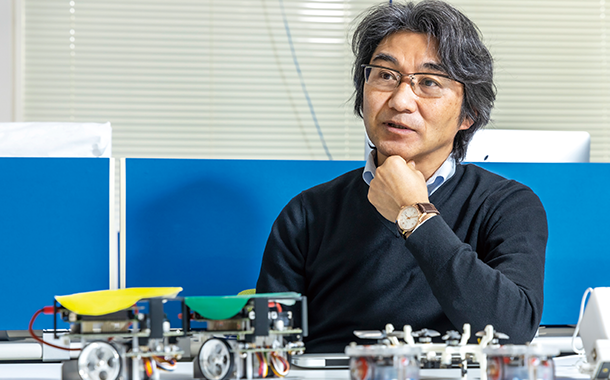
人やモノの位置を把握するシステム
具体的な研究内容を紹介してください。
研究の柱は4つあります。このうち、分かりやすい「自己組織型の屋内測位」と「群知能を使った移動センシングクラスター」について説明します。
自己組織型の屋内測位は、イベント会場や工場などで人やモノの動きを知るためのシステムです。最近、IoTやDX(デジタルトランスフォーメーション)の分野で、人やモノの位置の把握は非常に価値があると認識されています。例えば、ショッピングモールは、人の動線を管理してセールスプロモーションに活用したり、工場や建設現場では、安全管理のため人の動きを把握したり。また、病院や空港、イベント会場では、入院患者や搭乗予定客、来場者の位置を正確に知りたいなどの、多くのニーズがあります。
屋外ならGPSが利用できますが、屋内では決め手となるシステムがありません。電波を発する機器やカメラを使う方法ではたくさんの装置や頻繁なレイアウト変更が必要で、コストが掛かり維持管理が大変です。そのため、機器を設置しなくても人やモノの位置を特定するシステムを開発しました。
どのような仕組みになっているのでしょうか。
対象者のスマートフォンを利用する場合、どのスマートフォンが隣接しているかと、その隣接するスマートフォンが発する電波の強弱の情報をアプリでサーバーに送ります。サーバーに集まった情報から、全スマートフォンの隣接関係が分かり、これによりサーバー内で仮想的スマートフォンのネットワークを構成できます。ただし、スマートフォンの位置は不明ですので、スマートフォンの隣接関係のネットワークはランダムで未構造な状態です。このランダムなネットワークに取得したスマートフォン間の電波情報を繰り返し入力すると、未構造だったネットワークが自律して、ネットワーク構造を作り上げていきます。できた構造はネットワークのジオメトリ(形)を再現しており、ネットワークの形が分かるので、それを構成するスマートフォンの位置も分かることになります。つまり、何も設備がなくても、人が集まれば位置が分かることになります。このようにランダムで未構造な集団が、互いに相互作用し構造を作り上げていくことを自己組織化と言います。
ネットワークにおける自己組織化は、自然界や社会活動などあちこちで見られます。天体は重力で相互作用し、対数螺旋のネットワーク構造を作っています。また、人に置き換えると大学入学直後は友達関係がありませんが、次第に共振し合って人間関係のネットワークを構築していきます。同様に経済活動における企業間取引ネットワーク、国家間経済同盟、国家間軍事同盟も自己組織化の実例と言えます。
A system for capturing the positions of people and things
Tell us about the contents of your specific research field.
There are four pillars in our research. Of these, I will explain “self- organizing indoor localization” and “mobile sensing cluster using swarm intelligence,” which may be easy to understand.
Self-organizing indoor localization is a system for identifying the movements of people and things at an event site or a factory. Recently, in the fields of the IoT and Digital Transformation (DX), it has been recognized that it is very valuable to identify the positions of people and things. For example, a shopping mall manages the flow patterns of people for sales promotions and a factory or construction site monitors the movements of workers for safety management. In addition, there are a lot of needs at hospitals, airports, and event sites; for example, there might be a need to accurately know the positions of patients who are hospitalized, customers who will board an aircraft, and visitors who have visited an event site.
GPS is available at outdoor sites, but there is no decisive system available at indoor sites. If a method employs wireless devices and cameras, it is necessary to use many devices or to change the layout frequently, which may be costly and make the system difficult to maintain. For this reason, we have developed a system for identifying the positions of people and things without installing infrastructure equipment in advance for the positioning.
How does the system work?
When a target person uses their smartphone, a smartphone sends the following information to a server: which smartphone is located neighbor to the smartphone and the strength of radio signals received from the neighbor smartphone. From the information collected at the server, it is possible to identify the neighbor relationships of all smartphones, which makes it possible to establish a virtual network of smartphones on the server. However, the positions of the smartphones are unknown. Therefore, the network of the neighbor relationships of smartphones is random and unstructured. The information collected on the strength of radio signals between smartphones is repeatedly input to this random network. Through these repeated inputs, a structured network emerges autonomously from the unstructured network. In the structure created, the geometry (shape) of the network is reproduced, so it is possible to know the shape of the network. Therefore, it is possible to identify the positions of smartphones that compose the network. In other words, even if there is no infrastructure equipment for the positioning, it is possible to identify the positions of people when they gather. As I have described, a random, unstructured group gradually builds a structure by having individuals interact with one another, which is called self-organization.
Self-organization in networks can be seen here and there in the natural world, in social activities, and in other areas. Celestial bodies interact with one another via gravity, which creates a logarithmic spiral network structure. When this mechanism is applied to human beings, it can work as follows: people who have just entered a college have no relationships with friends, but they gradually resonate with other people and build networks of human relationships. Similarly, business-to-business transaction networks in business activities, economic alliances among nations, and military alliances among nations are also actual examples of self-organization.
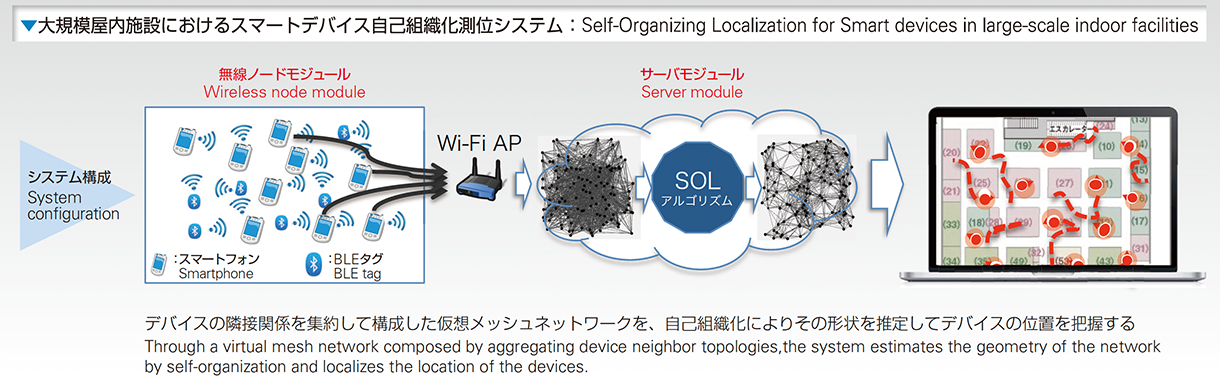
群れを分散させて要救助者を探す
次は、群知能を使った移動センシングクラスターについて教えてください。
魚や鳥の群れが知的な振る舞いをするのだから、ロボットを集めても同じことができるだろう、という発想から生まれた研究です。想定しているのは、地震などの災害によって人ががれき等に埋もれてしまった状況です。私たちは、体温を検知するセンサーなどを積んだ多数のロボットを使って探す方法を考えました。
例えば、50台のロボットで探すとします。1台が「この辺に居そうだ」という有力な情報を得た時に、数台が群れとなって近辺を重点的に探せば効率的です。そういう群れがあちこちにできるわけです。高性能な1台ではなく、簡素な機能ですがたくさんのロボットが群を形成する方が、柔軟で多様性・拡張性が高いと考えます。
Disperse clusters, and searching for people who need help
Next, tell us about the mobile sensing cluster that uses swarm intelligence.
This research was born out of the idea that a school of fish and a flock of birds act intelligently, so robots would also be able to act in the same way by working together. We assumed a situation where people are buried under rubble due to a disaster such as an earthquake. We came up with a method for searching for people using many robots equipped with sensors that can detect human body temperatures.
For example, suppose you search for a person using 50 robots. When a robot gets promising information, such as “a person might be buried around here,” it is efficient for several robots to search the neighborhood intensively together as a cluster. Such clusters would be created here and there. We think that a method for collecting information using many simple functional robots is more adaptable and scalable than a method using a single high-performance robot.
柔らかいネットワークは「生きている」
ネットワークって面白いですね。
多様性を内包したネットワークは、状況の変化に対応できます。人間にもいろいろな人がいて、こういう時に活躍するグループ、別の場合に活躍するグループと集まる人によって活躍の場面が異なるグループは自然にできあがりますよね。それと同じです。
情報処理の世界には、最適化や収束を美徳とする考え方があります。しかし、特定の状況に最適化してしまったネットワークは変化に対応できず、生命体としては死滅することになります。私たちは状況が変わるものに適時適応するため、ネットワークにおいて、収束させることなく変遷させ、最適化して選択肢を狭めるのではなく、多様性を内包するしなやかで生命体のような構造を作り出すことを目指しています。
ただ、生命体を模したメカニズムは、時として判断を間違えることがあるんですよ。また、実際に動かしてみないとどのような構造を作り出すか分かりません。柔軟な構造を保ちながら、間違いをどれだけ抑えるか。それが工学応用をする上で最も重要な課題で、面白さを感じる点でもあります。
A soft network is "alive"
Networks are interesting, are they not?
A diversity-encompassed network can respond to changing situations. Human beings are diverse; for example, a certain group might play an active role in a certain situation, while another group might play an active role in another situation. In such a way, a group that plays an active role in a different situation is created spontaneously depending on the people who have gathered together. A diversity-encompassed network is the same.
In information processing, there is a similar thought that optimization and convergence should be considered as virtues. However, a network that has been optimized for a particular situation cannot respond to changes and will die like a living organism. To adapt to changing situations at the right time, in a network, we aim to create a structure like a living organism via diversity and encompass flexibility by having the network change without making it converge, instead of optimizing it by narrowing down choices.
However, a mechanism that imitates a living organism sometimes makes errors in judgment. In addition, we don't know what kind of structure the mechanism will create until the mechanism is actually operated. To what extent can the mechanism eliminate mistakes while maintaining a flexible structure? This is the most important issue in engineering applications. It is also a point that interests us.
社会で使われる技術を開発しよう
研究室のモットーは何でしょうか。
研究のための研究では面白くありません。実際に社会で使われる技術を開発しよう、という目標を掲げています。そのため、技術展示会に出向き、いろいろな企業と接触するようにしています。学会での議論は深いけれど狭い。展示会では考えもしなかった意見を聞くことができ、視野が広がります。展示会で刺激を受け、研究の方向性を変えようかと検討することもあります。
自己組織化や群知能は、自然界や人間の社会活動において見ることのできるメカニズムであり、構造を作り上げて、構造を変えていく深遠なテーマとして考えています。そしてこれらに共通する振る舞いは変遷と多様性です。私たちは最適化や収束ではなく、この変遷と多様性を求める研究をしているのです。
Developing technologies that can be used in our society
What is the motto of your laboratory?
Research is not interesting if the goal is just to carry out research. The target we have set is to try to develop technologies that will be used in our society. Therefore, we try to visit technology exhibitions and contact various companies. Discussions at academic conferences are deep, but narrow in scope. At an exhibition, we can hear opinions from various people that we had never considered, and we can broaden our perspectives. There are also cases where we gain inspiration from an exhibition and consider whether or not to change the orientation of our research.
Self-organization and swarm intelligence are mechanisms that can be seen in the natural world and in the social activities of human beings, and we think of them as profound themes for creating a structure and then changing that structure. Actions common to them are transitions and diversity. We have been conducting research that searches for these transitions and diversity, not for optimization and convergence.
複数のドローンにより多群を形成して数や場所が不明のイベントを探索
Search for unknown events by forming multiple swarms with multiple drones.
関西大学YouTubeチャンネル